News
Training Artificial Intelligence Models in Uali
At Uali, we have developed a robust approach to training artificial intelligence (AI) models, which powers our remote monitoring solutions in the energy industry. We currently have a variety of in-house developed AI models designed to optimize the operation and safety of energy assets.
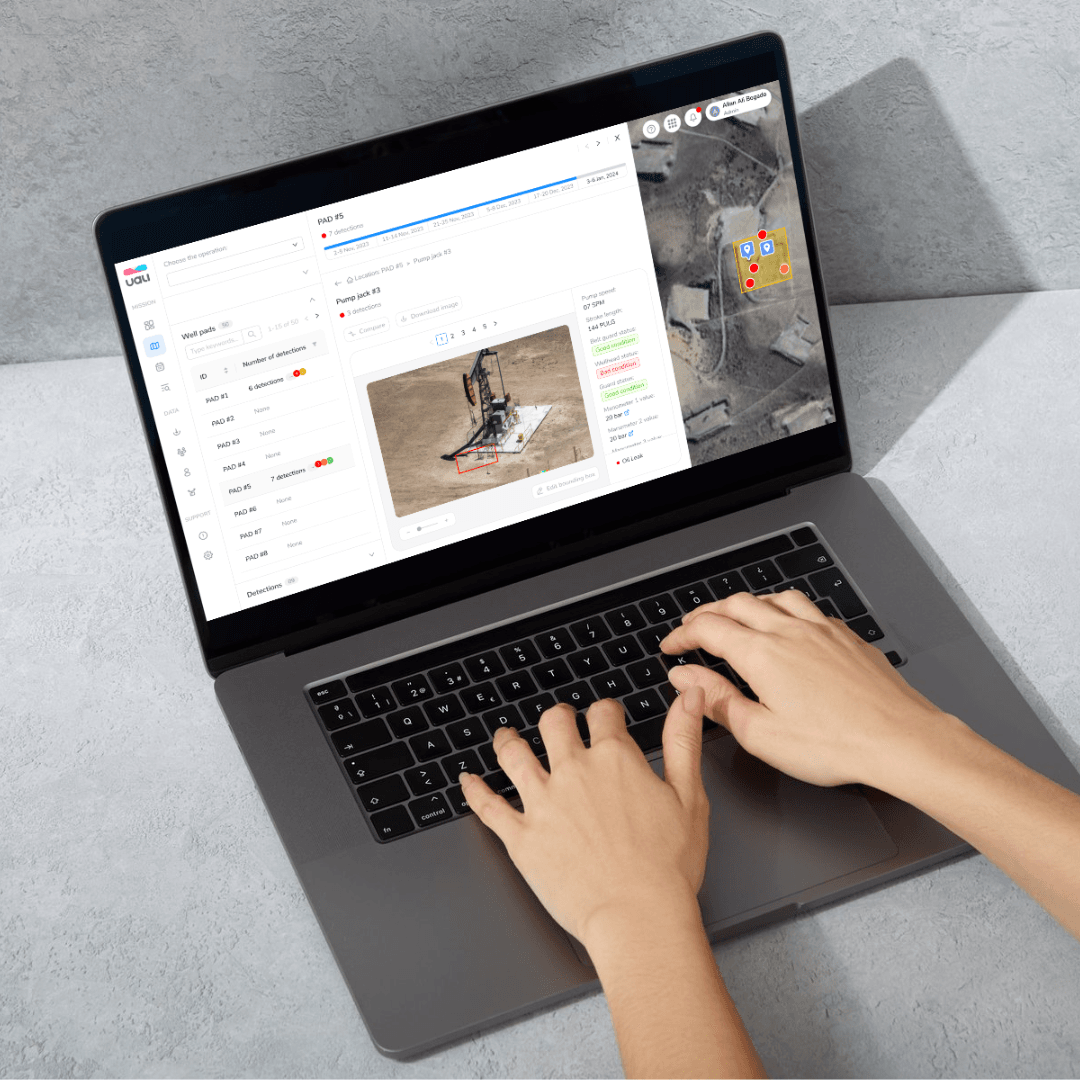
Model Training Methodology
Training an AI model involves several stages that ensure its ability to detect objects and generate accurate results. At Uali, our AI models are designed to adapt to the specific challenges of the energy industry, providing scalable and efficient solutions that continuously improve with each training cycle.
1. Problem Definition
The first step for a robust model is to identify the type of objects to be detected. For example, it could be segmenting vehicles in a field or identifying anomalies in wind turbines. In addition, drone missions are planned to obtain images from optimal perspectives for processing.
2. Data Collection and Preparation
A good dataset is essential.A large set of useful photos is required, at least 1,000 to obtain a preliminary model. The more representative photos of the object or scene to be detected, the better the model's performance will be.
3. Data Annotation
This stage involves manually labeling the photos, drawing "bounding boxes" (feedback module) around the objects of interest. It is a laborious but critical process to teach the model to correctly detect the elements.
4. Preprocessing and Simulations
Images undergo adjustments such as pixel value normalization and resizing, ensuring their compatibility with the model architecture. If data is insufficient, objects can be simulated to enrich the dataset. For example, oil spill images were initially generated by superimposing real spills on uneventful photos.
5. Data Splitting
The dataset is split into three sets: training, validation, and testing. This separation ensures that the model learns correctly and is validated on unseen data, evaluating its real-world performance.
6. Model Training
The model "learns" by repeatedly processing the annotated dataset. It gradually improves its ability to detect the desired objects, adjusting its parameters as it sees more examples.
7. Model Evaluation
After training, the model is evaluated on images not used during the learning phase to verify its accuracy. If the performance is not optimal, the training time is adjusted or the dataset is expanded.
8. Inference and Logic
Once trained, the model is ready to perform inferences, that is, process new images and detect objects. In addition, additional rules are applied to generate alerts or actions based on the results obtained.
9. Deployment
The model is integrated into our production platform, automating image processing every time new mission photos are uploaded. The results are ready to be validated by our clients on our energy asset management platform.
10. Maintenance and Continuous Improvement
The work doesn’t end with deployment. Models require constant maintenance and improvement. Collection of new data and expert validation allow the model to be recalibrated, improving its accuracy and adaptability over time.
At Uali we combine drones with artificial intelligence to provide accurate and actionable data to the energy industry, facilitating decision-making in the care of their assets and thus generating a positive environmental impact. Get to know us!
Giselle Balzano
Product Manager